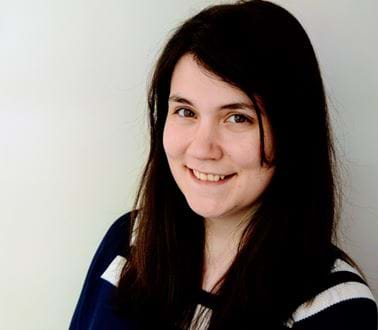
Roke meets
Angharad
Our data science team, led by Head of Profession Angharad, creates capability for extracting valuable insight from data by designing and developing data-driven systems and algorithms, making data accessible, manageable, and useful to customers. We sat down with Angharad to discuss her career in the industry, find out more about how the data science team are helping customers get the best from their data and discuss how their work dovetails with the rest of our professions.
Tell us about your entry into the industry
When I undertook a degree titled ‘Astrophysics and Smart Systems’ people often asked me what I was going to do with that, and to be honest, I didn’t have an exact plan – I just wanted to learn about the things I found interesting! After university I got in to ‘Big Data’ as a technical trainer, mostly focusing on Hadoop-based technologies with a sprinkling of enterprise architecture, Python, and databases. I then decided to move into engineering to grow my practical skills and since joining Roke have worked on projects spanning software engineering, cloud, Big Data, and machine learning challenges.
How has Roke helped develop your career as an engineer?
Being able to work on a variety of different projects with a range of teams has really helped develop my technical and interpersonal skills. From writing reports and developing proof-of-concepts, to long-running software engineering projects, it’s a great environment to learn lots of new skills and ways of working. Since joining, I’ve been involved in innovation, bidding, and engineering, and have grown to take on the role of head of profession in order to steer the direction and give guidance in the area of data science.
Tells us about the main principles of data science
To preface this: ‘Data science’ and ‘Big Data’ have, unfortunately, become buzz-phrases that can mean lots of things to lots of different people. Search for it on the internet and you’ll get a range of overviews.
Engineers within the Data Science profession at Roke focus on areas including data engineering, data analytics, batch and stream processing, and data visualisation. What do we mean by this?
- Data engineering includes creating architectures for storing, processing, and distributing data, looking at the entire data management lifecycle, and DataOps – taking DevOps techniques from software engineering and applying them to data-driven platforms
- Data analytics focuses on traditional methods of querying data in order to derive insights or inform future decisions
- Batch and stream processing look at how we can work with large amounts of data, either in a historical format (batch) or live (stream)
- Data visualisation aims to understand the optimal methods for displaying information in order to demonstrate insight.
Data science requires skills in maths, computer science, and domain knowledge in order to deliver. There’s also a key element of understanding differences between structured (e.g. rows and columns in a database) and unstructured data (e.g. images or unstructured text).
How does data science overlap with our other professions? Particularly in AI & machine learning.
We work closely with the AI&ML and software professions to deliver solutions that provide insight to data, informing business decisions. We work with software to capture, transform, store, and present data so that end-users can access and query data efficiently. We work with AI&ML to find the best solution for analysing, querying, predicting, and classifying data. Before data can be used effectively for training or testing a machine-learned algorithm, it should be properly cleansed, processed, and stored. Effectively presenting the outputs of that algorithm is also key to delivering success, so data science and AI&ML are closely linked in the lifecycle of gaining insight from data.
What are we doing at Roke that is at the forefront of data science?
At Roke, we are always looking at new technologies and research in the areas relevant to our profession. A key area of development for us in data science at the moment is the use of cloud technology and microservices to scale data pipelines that can cope with increasing amounts of data. We also look for novel methods that reduce the burden on human operators, such as triaging data to condense the information presented to the end user.
What does the future of data science look like to you?
I’m consistently astounded at the ever-increasing amount of data that society produces. We must continue to develop novel ways of storing, processing, and presenting that data in a useful way. Summarising and searching data effectively are core to this, but so are ‘Big Data’ technologies that aim to use all of the data available. Having the experience to determine which needs to be used in a given scenario will be key, and as we transition towards cloud-native delivery, when to run data pipelines on premise or in the cloud will be an important question to answer. Creating repeatable and scalable infrastructure through DataOps techniques will become increasingly important in order to configure, create, and maintain large-scale pipelines.