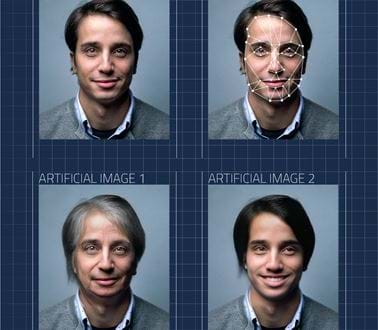
Deepfakes
Improved technology and the always-online world we live in means it’s becoming increasingly more difficult to judge if the media we consume is sincere or genuine. Deepfakes, which are synthetic or fake videos that are generated using artificial intelligence, are a common sight on the internet. Many focus on switching faces or facial expressions to deceive the viewer and spread misinformation.
The main method for creating a deepfake involves the training of a generative adversarial network (GAN). This is a type of machine learning (ML) framework where two neural networks compete against each other. One network (a generator) creates deepfaked video candidates and the other network (a discriminator) tries to classify the candidates as either real or fake.
The two networks improve by competing against each other, with the generator getting better at creating fakes and the discriminator getting better at spotting them.
Based on this technology deepfakes are becoming increasingly sophisticated and harder to distinguish from real videos. Additionally, commercial tools are making it easier for anyone to make them (even if they have no ML experience).
How deepfakes can be dangerous
Deepfakes first gained notoriety following a Reddit post outlining how to create them, leading to a glut of falsified videos being created and shared on the platform. Today, access to that particular thread on Reddit has been blocked, but it’s now even easier to access countless apps and tutorials on a range of websites.
Researchers have found that falsified news online is likely to spread faster than accurate information. They speculate this is because there is a bias to sharing negative news over positive news. Falsified news or fake videos are more likely to meet this criteria and therefore are more likely to be shared.
The potential for this technology to spread misinformation across a multitude of areas is vast. Whether through impersonating someone’s voice to commit fraud and fool a colleague, family or friend into transferring money, or damaging the reputation of a business by falsely creating a video from a CEO announcing a major financial loss, or the termination of a partnership with another company.
So what can we do about it?
One option in tackling the deepfake problem involves researching ways to detect deepfakes using ML algorithms. Many deepfakes are created by improving the generation algorithm in sync with the detection algorithm. This can mean that improvements in detection algorithms are quickly followed by improvements in generation algorithms. For example; for a while it was possible to spot a deepfake by tracking the speaker’s eye movements, which tended to be unnatural. Shortly after this method was identified, algorithms were tweaked to include better blinking. It means that these algorithms are creating somewhat of an arms race between creators and detectors. But if researchers can keep finding ‘tells’ in online media, then creators will be forced to continually develop new algorithms.
It’s also possible to add a signature to a video to prove authenticity. Much like watermarks are used on official documents to mark them as legitimate, adding a signature to videos would make it difficult for deepfake systems to tamper with these. Traditionally, incorporating these signatures into live video has been challenging due to variable streaming bit rates, but our innovation teams have recently developed a feature based authentication measure that can be attached to video streams as a signature. It’s informed by visible elements of the footage, before being generated through ML techniques. Viewers or third parties can then authenticate videos by executing the same algorithm and comparing signatures.
The future of deepfakes
Many companies are now providing new and improved methods for detecting deepfakes, but, as is to be expected, the technology used to create them is also evolving. Falsified news isn’t going to disappear overnight, and one of the best things we can do is arm ourselves with the knowledge of what it is and what tools we do have to spot it.
Recently Microsoft announced two different tools for combating deepfakes; a video authenticator (a tool for analysing videos and still photos to generate a manipulation score) and a video authenticity prover (made up of a tool to generate a certificate and a hash for a video, and a tool to check them). But these tools are currently only available to journalists and politicians, and are still in their infancy. A range of parties have been involved in developing these tools - a sign of how difficult it is to develop these things in isolation, even for a huge company like Microsoft.
Moving forward
Uses for deepfakes aren’t all negative however. In fact, a number of sources have reported that they’re being adapted innovatively to provide a host of positive solutions, including within medicine, entertainment and education. It will be interesting to see the future benefits this technology could bring when used responsibly.
Tackling the spread of misinformation and deepfakes requires a group effort, and the collaboration of minds from tech companies, lawyers, law enforcement and the general public is invaluable.
At Roke, we are constantly trying to find new ways to enable customers to access and contribute to our innovations. It’s only by establishing collaborative working practices, such as our Innovation Hub, that we can keep up with the pace of change of technology and proactively make use of that change, instead of merely having to react to the threats that it brings.
Related news, insights and innovations
Find out more about our cutting-edge expertise.
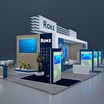

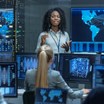